The Blending of Mathematics and Polymers in Shaping the Future of Plastics
Mathematics and Polymers seems two different fields but the combination of both is necessary to advancement in plastics and polymer industries.
Without having entered a laboratory, scientists may use mathematics to predict the behaviour of polymers.
Using statistical mechanics, differential equations, and computational simulations, researchers can model polymer chains, interactions, and behaviour under stress or heat. These models help us to answer questions like:
• How flexible is this polymer supposed to be?
• Will a certain environment allow it to biodegrade?
• How strong is it under pressure or pulling?
By solving equations that describe molecular motion and energy distribution, we can simulate the behaviour of polymers in real life.
Modern mathematics isn’t just about pen-and-paper equations. Large datasets of polymer structures and properties may now be analysed by identifying trends and forecast outcomes thanks to the development of machine learning and data-driven models. This combination is highly effective these days for real-world problems such as Biodegradable plastics, Recyclable materials, various applications like automotive industry, aerospace, medical, etc.
The mixture of polymers with mathematics is more than just a theoretical exercise. It is a powerful tool that is changing the way we design, produce, and use plastics. We are getting closer to a future with smarter, safer, and more sustainable plastics by applying mathematics to find out the complexities of molecular structure.
The integration of mathematics and polymer science holds tremendous potential for shaping the future of plastics, yet it comes with a host of complex challenges. One major difficulty lies in accurately modeling the intricate behavior of polymers, which exhibit nonlinear, time-dependent properties such as viscoelasticity and creep. Capturing these behaviors mathematically requires advanced equations and often leads to computationally intensive simulations. Adding to this complexity is the challenge of multiscale modeling—linking atomic or molecular level interactions to macroscopic behaviors is far from straightforward, yet essential for designing plastics that perform predictably in real-world applications.
Another significant hurdle is the limitation of current computational models, which often require simplifying assumptions that compromise accuracy. Developing new algorithms that can simulate large, complex polymer systems more efficiently remains a key research focus. Furthermore, as sustainability becomes a top priority, there is a growing need for mathematical models that can predict the environmental impact of various polymers, including their biodegradability, recyclability, and overall lifecycle. This adds another layer of complexity, as such models must integrate both chemical behavior and environmental variables.
Designing novel polymers with specific properties presents another challenge. While inverse design and optimization techniques show promise, they require robust mathematical frameworks that can accurately map structure to function. The integration of machine learning with traditional physics-based models offers new opportunities but also introduces difficulties in balancing accuracy, interpretability, and generalizability. In industrial contexts, simulating manufacturing processes such as extrusion or injection molding in real-time demands highly efficient and precise mathematical models, which can be difficult to achieve given current technological constraints.
-Mr. Praharsh Patel, Asst. Professor, School of Engineering, Plastindia International University.
Similar Posts:
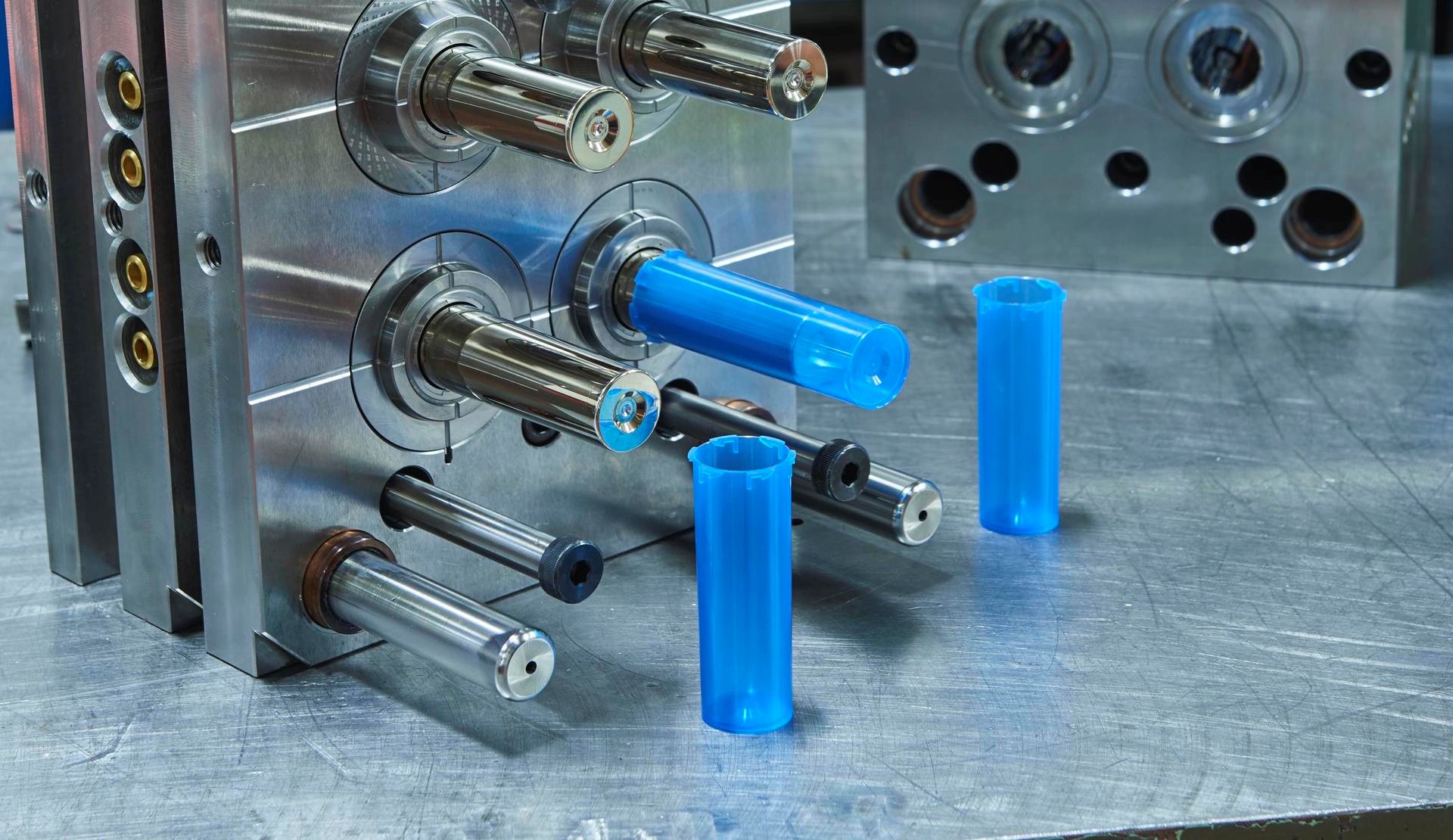
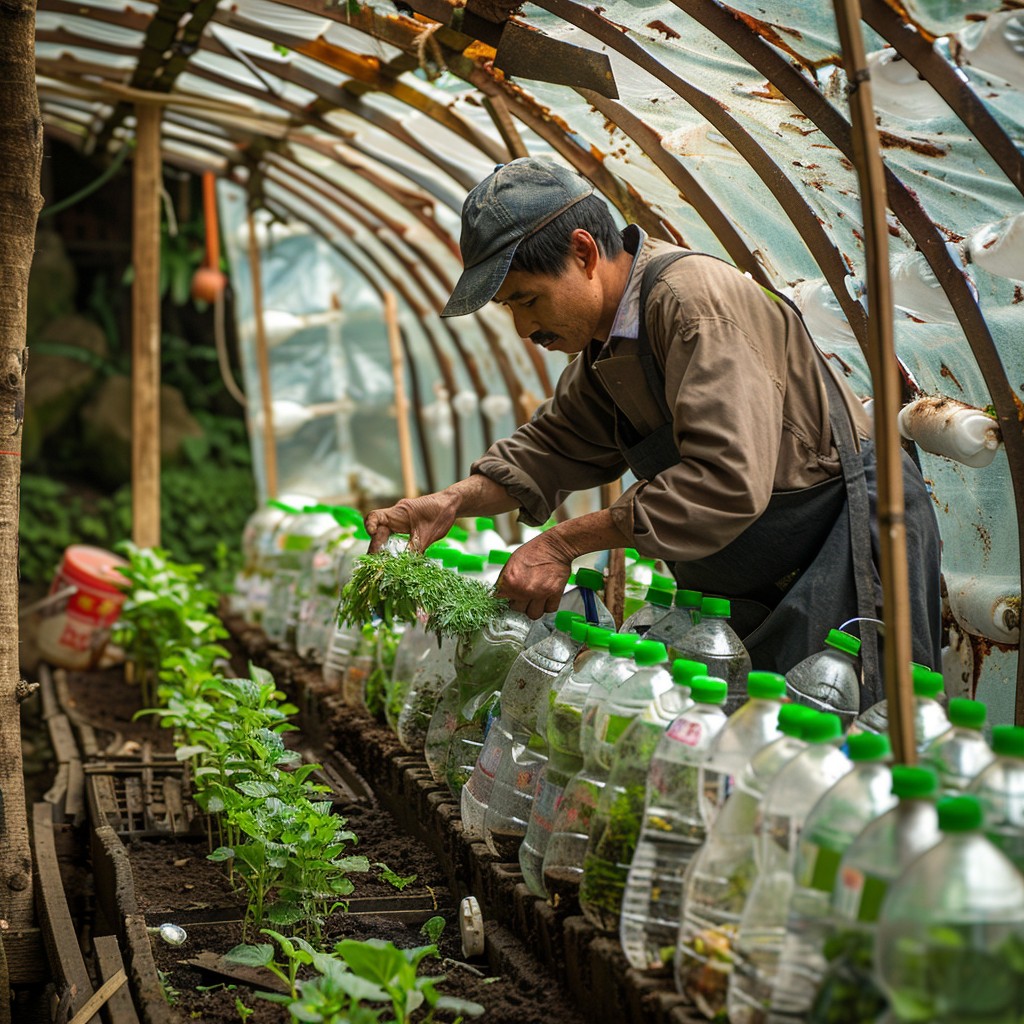
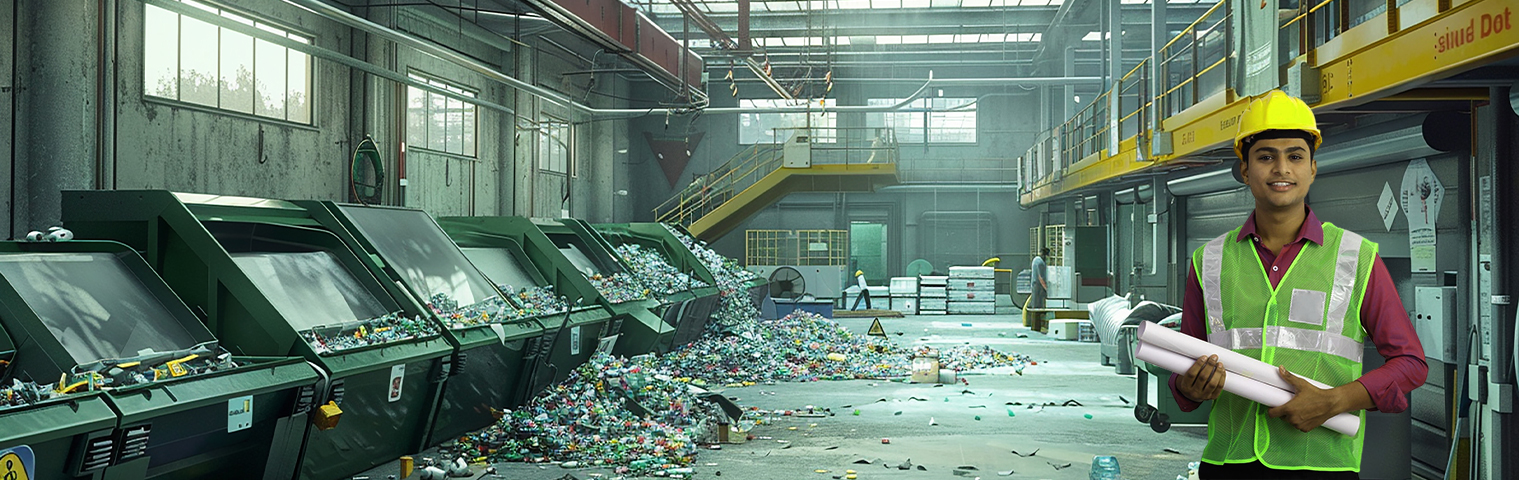
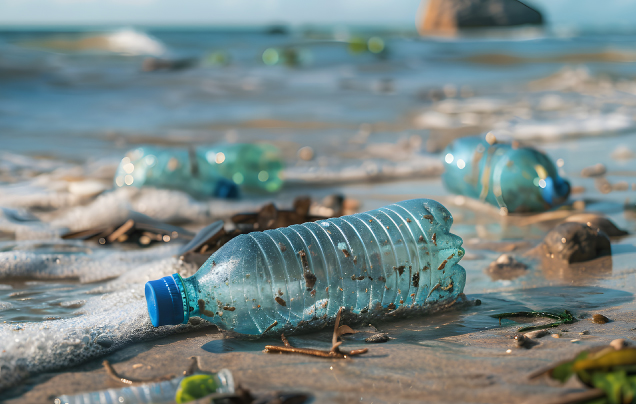